The importance of data management and governance continues to grow with the rise of generative AI and the ever-increasing volume of data. Effective Data Quality Management (DQM), supported by Adaptive Data Governance (ADG), is more critical than ever and should be a central goal for data-driven organizations.
The landscape of data governance and its best practices are evolving rapidly. New regional data privacy laws and consumer demands for transparency have made clear and consistent practices a necessity. For many organizations, implementing data governance is a defensive response to new regulations. However, good data governance offers much more than just compliance; it can enhance efficiency, collaboration, and foster a culture of data-driven decision-making.
Generative AI represents a dramatic shift in how data is collected and used. Companies will need firm yet flexible data policies to effectively respond to the rapid changes brought about by new technologies. Robust DQM combined with adaptive data governance policies ensures that decisions are based on high-quality information while creating a framework that allows for near-unlimited adaptability and flexibility.
The High Costs of Poor Data
Insufficient Data Quality Management (DQM) can have costly consequences. According to Gartner, poor-quality data costs companies nearly $10 million annually; in the U.S. alone, the costs of bad data exceed $3 trillion per year. Poor data directly impacts the bottom line; for nearly 90% of U.S. companies, this translates to an average revenue decline of 12%. These costs stem from various effects, including reputational damage, missed opportunities, low data utilization due to lack of trust, high data debt, and increased costs and inefficiencies in data processing.
However, for DQM to be effective, especially on a large scale, it must be guided by Data Governance (DG). Data governance refers to the broad strategic philosophy of an organization for managing, using, and securing data. It not only defines the policies for handling data within the organization but also outlines the people involved, their roles and responsibilities regarding data, and the core principles underpinning the strategy.
Unfortunately, fewer than 50% of organizations have implemented such a philosophy. Among those that have, efforts are often focused on regulatory compliance and little else. This defensive approach to DG concentrates on cleaning, qualifying, maintaining, and securing data, and while this approach provides essential safeguards, it often limits agility and decision-making complexity.
A DG model that promotes a proactive (or offensive) approach can go beyond compliance and help organizations target specific business goals or employee behaviors. Well-designed DG can be applied across the entire operational spectrum to achieve objectives such as ensuring information security, complying with evolving data privacy regulations, developing data literacy, maintaining fine-grained access permissions, and promptly responding to information requests.
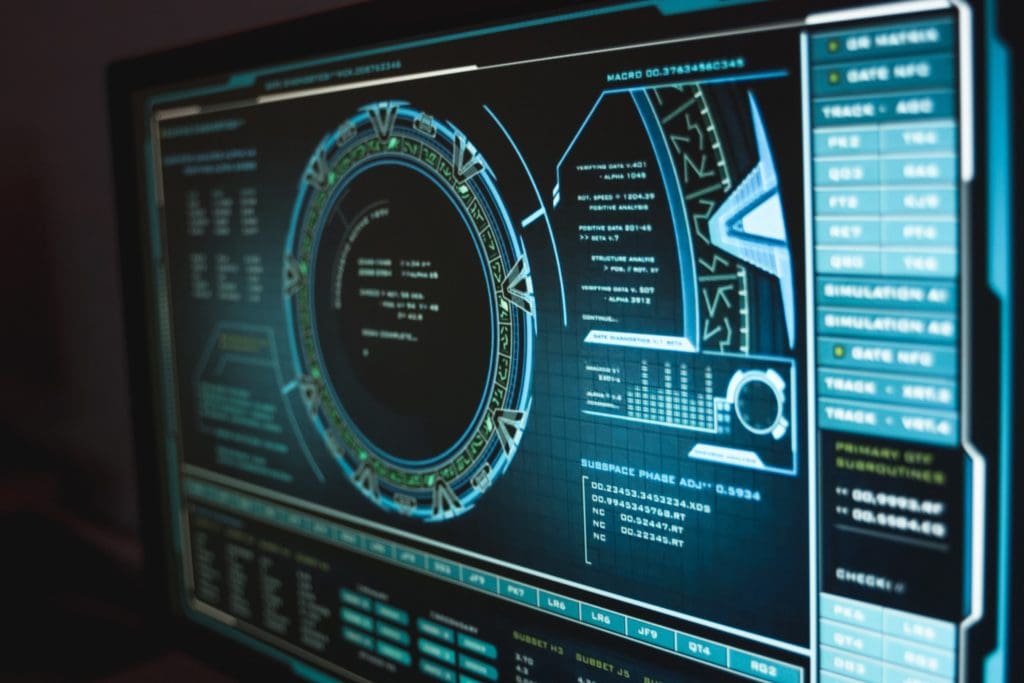
Adaptive Data Governance
Lean, adaptive data governance (ADG) offers an alternative to defensive, control-based strategies. Instead of a top-down, one-size-fits-all data framework, ADG takes a more proactive and collaborative approach to creating clear guidelines that enable rather than restrict data usage. Data processes are designed not only to ensure compliance but also to align with and evolve alongside organizational goals and priorities. With the flexibility to adapt data processes for specific purposes and use cases, lean methods provide decision-makers with opportunities that are not possible with more restrictive defensive stances.
Fair or not, data policies are often seen as disruptive to existing procedures, adding extra work with little perceived value, and non-compliance can easily derail carefully designed data processes. By taking a collaborative approach to policy design, ADG gives data consumers a direct voice in crafting flexible guidelines that can be adapted to various departments and contexts. Defining clear roles and responsibilities for data owners, stewards, and users, and integrating governance into existing workflows, reduces conflict and non-compliance, fostering a data-driven culture.
While regulatory compliance may not be the only aspect of DG, it remains crucial. With significant new and updated privacy laws in Europe and North America in 2023, a recent report shows that only half of U.S. and U.K. organizations feel prepared to meet the new requirements. The flexibility at the core of ADG enables quicker responses to regulatory changes and smoother adaptation to different local laws within multinational companies.
ADG and AI
The rise of generative AI, spearheaded by the launch of OpenAI’s ChatGPT in November 2022, represents a radical shift in business operations, with McKinsey estimating that generative AI could add $2.6 trillion to $4.4 trillion annually to the global economy. The publicly accessible version of ChatGPT attracted an unprecedented one million users in just five days and reached 100 million in two months. OpenAI has also announced significant partnerships with clients like Morgan Stanley and the government of Iceland, and a variety of other AI services such as Bard, Claude, GitHub Copilot, and Stable Diffusion have followed ChatGPT to market, offering new automation opportunities and the potential for increased productivity and reduced costs.
As generative AI tools open up revolutionary new ways to utilize data, the methods by which organizations collect, handle, evaluate, and conceptualize data will also change. AI governance is not only necessary to ensure compliance and achieve a better ROI for new technologies but also to uphold the company’s values and ethics. As AI’s role within social and regulatory structures continues to evolve, agility in designing and implementing policies and processes will be critical to navigating the AI revolution successfully.
With new technologies, the old adage “garbage in, garbage out” still holds true; reliable, effective AI cannot be built on a foundation of poor data. Therefore, parameters must be set to measure the reliability, accuracy, and quality of input data. To prevent AI algorithms from becoming “black boxes,” it is crucial to establish robust guidelines for their use and monitoring, such as requiring audit trails and setting regular review and iteration cycles. Additionally, the outputs of new AI processes must be monitored to detect bias or “hallucinations” and to maintain quality over time. An agile approach to AI governance will help organizations maximize AI’s value, and adaptability will be essential to addressing emerging risks and best practices.
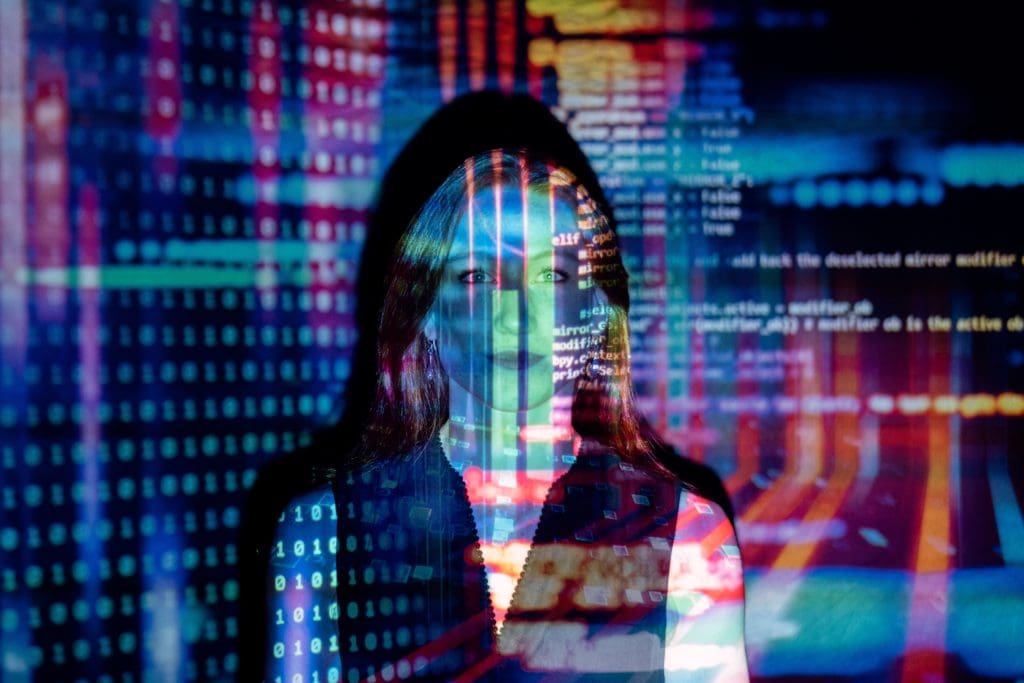
One Size Does Not Fit All
A multi-layered governance approach succeeds for the same reasons as any other policy or process—it is relevant and useful. This all hinges on transparent, logical data policies, responsive feedback mechanisms, and user-friendly technologies. The right combination of these factors increases compliance within the organization and ensures that decisions are made with risk awareness and security in mind.
Shifting to Adaptive Data Governance (ADG) takes time; it requires a radical redesign of an organization’s philosophical approach to data and analytics. While the right software is essential for implementing any data policy, ADG is just as much about operations, security, risk management, employee experience, and customer engagement as it is about technology. The good news is that this framework follows a lean approach, and the best way to achieve significant changes with ADG is to start small and build from there.
Guide to Implementing Adaptive Data Governance (ADG)
Adaptive Data Governance (ADG) enables companies to adapt to changing business conditions and requirements by designing flexible data strategies and solutions. This approach is especially useful in rapidly evolving industries and environments. Here’s a basic guide to implementing adaptive data governance:
1. Assess the Current State
– Analyze your existing data infrastructures, systems, and processes.
– Identify strengths, weaknesses, opportunities, and risks in your current data management strategy.
2. Define Business Goals
– Set clear business objectives and requirements that adaptive data governance aims to achieve.
– Consider both short-term and long-term perspectives.
3. Technology Assessment
– Explore available technologies and tools that support flexibility and adaptability in data management.
– Prioritize solutions that offer scalability, interoperability, and easy integration.
4. Data Modeling
– Develop adaptive data models that can accommodate changes in business requirements.
– Utilize techniques like data abstraction and modular data architectures.
5. Leverage Automation
– Implement automation tools to streamline routine tasks such as data synchronization, cleansing, and integration.
– Consider using machine learning and AI tools to promote adaptive processes and facilitate data-driven decision-making.
6. Establish a Feedback System
– Create mechanisms to gather regular feedback from stakeholders and end-users.
– Use this feedback to continually review and adjust your data management processes.
7. Training and Education
– Invest in training your team to ensure they can effectively use adaptive data governance tools and processes.
– Foster a culture of lifelong learning and adaptability.
8. Data Privacy and Compliance
– Ensure your adaptive data governance strategy complies with applicable data privacy laws and regulations.
– Implement security protocols and policies to safeguard the integrity and security of your data.
9. Continuous Review
– Schedule regular reviews and audits of your data governance strategy and processes.
– Adapt your approaches based on technological advancements, business needs, and feedback.
By following this guide, companies can implement adaptive data governance that is both responsive and proactive in reacting to changes in the business environment. This approach allows them to remain competitive and harness the benefits of data in an ever-changing world.